Is ML Ops the Key to Enhanced Efficiency in Machine Learning Processes?
Dive into the transformative role of ML Ops in machine learning lifecycles and discover how it elevates efficiency and streamlines processes. Learn the benefits and future trends with Antematter's expert insights.
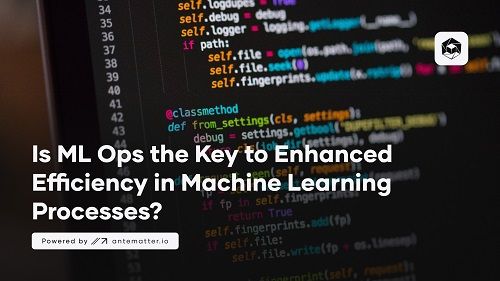
In the realm of machine learning, the integration and operationalization of models into production systems are just as crucial as their development. This is where ML Ops (Machine Learning Operations) comes into play, marking its significance in the current machine learning landscape. ML Ops, a compound of "Machine Learning" and "Operations," is increasingly recognized as a pivotal element in enhancing the efficiency and effectiveness of machine learning processes. It bridges the gap between the model development and operational stages, ensuring a seamless transition from one to the other.
The primary focus of ML Ops is to streamline and automate the machine learning lifecycle, which encompasses everything from data preparation to model training, deployment, monitoring, and management. By adopting ML Ops, organizations aim to optimize their machine learning processes, thus achieving greater "Machine Learning Efficiency" and operational effectiveness.
As we delve into this topic, we aim to explore and answer a pressing question in the AI and data science community: Is ML Ops the key to enhanced efficiency in machine learning processes? This query probes not only the technical aspects but also the broader impacts of ML Ops in the ever-evolving field of machine learning.
The Evolution of ML Ops
The journey of ML Ops traces back to the early days of machine learning, where the primary focus was on model development. As machine learning models became more complex and data-intensive, the need for a streamlined process to deploy these models into production became evident. This necessity gave birth to ML Ops, an interdisciplinary approach that combines "Data science automation," "Machine learning scalability," and "DevOps for machine learning" practices.
Initially, the deployment of machine learning models was a cumbersome process, often plagued with inefficiencies and a lack of coordination between data scientists and IT teams. The evolution of ML Ops has been largely driven by the need to overcome these hurdles and to ensure that machine learning models are not only developed efficiently but are also seamlessly integrated into operational environments.
Today, ML Ops stands at the convergence of machine learning and operations, incorporating best practices from both worlds. It emphasizes the importance of continuous integration and continuous deployment (CI/CD) in machine learning, mirroring principles from traditional software development. The incorporation of "AI model deployment" and "Automated machine learning workflows" into ML Ops has been pivotal in this evolution, enabling faster and more reliable model deployment.
A key milestone in the evolution of ML Ops has been its focus on scalability. As machine learning applications have grown in complexity and size, ML Ops has evolved to ensure that these systems can scale effectively, addressing the challenges of "Machine learning scalability." This aspect is crucial in a business context where the volume of data and the need for rapid decision-making constantly expand.
The integration of "Data science automation" into ML Ops has streamlined the entire machine learning lifecycle, reducing the time and resources required to bring models from conception to production. This integration has not only increased efficiency but also improved the reproducibility and reliability of machine learning workflows.
ML Ops: Enhancing Machine Learning Efficiency
The role of ML Ops in boosting the efficiency of machine learning processes is increasingly recognized in the data science community. By integrating practices from "Data science automation" and "DevOps for machine learning," ML Ops significantly streamlines the machine learning lifecycle, from development to deployment and monitoring.
A central aspect where ML Ops shines is in "Optimizing Machine Learning" processes. It enables data scientists and engineers to focus on innovation rather than operational challenges. By automating routine tasks and streamlining workflows, ML Ops reduces the time-to-market for machine learning models and improves the "Machine Learning Efficiency" of organizations.
Recent studies highlight the impact of ML Ops in reducing the operational overhead associated with machine learning. For instance, the adoption of "Continuous integration in machine learning" and "Automated machine learning workflows" significantly decreases the manual effort required in model updating and maintenance. This automation is particularly beneficial in dynamically changing environments, where models need frequent updates to stay relevant.
Moreover, ML Ops facilitates "Machine learning scalability" by providing a framework to manage and deploy models at scale. This scalability is crucial for businesses dealing with large volumes of data and complex models. By leveraging scalable architectures and efficient resource management techniques, ML Ops ensures that machine learning systems can handle growing data volumes and computational needs without compromising performance.
"AI model deployment" is another area where ML Ops contributes significantly. It ensures that the transition of a machine learning model from a development environment to a production setting is smooth and reliable. This process involves not only deploying the model but also setting up monitoring and logging mechanisms to track its performance in real-time, enabling quick identification and resolution of any issues.
Incorporating "ML Ops in Machine Learning" processes also leads to enhanced "AI operational efficiency." It aligns the objectives of data science and IT operations teams, fostering a collaborative environment where both the development and operational aspects of machine learning are given equal importance. This alignment is key in achieving a seamless and efficient machine learning lifecycle.
Technical Deep-Dive: How ML Ops Optimizes Machine Learning Lifecycles
ML Ops stands at the forefront of revolutionizing machine learning lifecycles, significantly enhancing "Machine Learning Efficiency" and operationalizing AI models. This technical deep-dive explores how ML Ops integrates various functions, including "Continuous integration in machine learning," "AI model deployment," and "Machine learning scalability."
Continuous Integration and Delivery (CI/CD) in Machine Learning:
ML Ops adopts the principles of DevOps, applying them to machine learning. "Continuous integration in machine learning" ensures that new code changes are automatically tested and integrated, reducing the development cycle and increasing the release rate of new models. This automation streamlines the process of "Optimizing Machine Learning," making it more agile and efficient.
AI Model Deployment and Management:
Deploying AI models into production environments is a critical phase where ML Ops plays a pivotal role. It involves not just deploying the model but also monitoring its performance, scaling it according to demand, and updating it as needed. "AI model deployment" and "ML model management" are thus essential components of ML Ops, ensuring that models remain accurate and efficient over time.
Scalability and Resource Management:
One of the key "ML Ops Benefits" is its ability to handle "Machine learning scalability." As the amount of data and the complexity of models increase, ML Ops provides the framework to scale these models efficiently. This includes allocating resources dynamically and ensuring that the infrastructure can handle the increased load without compromising performance.
Automated Machine Learning Workflows:
ML Ops automates the entire machine learning workflow, from data preparation and model training to validation and deployment. This automation, part of "Data science automation," minimizes manual intervention and accelerates the model development cycle, contributing to the "AI operational efficiency."
Data Orchestration in ML:
Effective data management is a cornerstone of successful machine learning projects. ML Ops facilitates "Data orchestration in ML," ensuring that the right data is available at the right time, and is processed efficiently. This orchestration is critical in managing complex data pipelines and maintaining data quality throughout the lifecycle.
Integrating ML Ops into Existing Systems:
A major aspect of "ML Operationalization" involves integrating ML Ops into existing IT and data environments. This integration requires a strategic approach, ensuring that ML Ops tools and processes are compatible with existing systems and can leverage them effectively.
ML Ops in Action: Case Studies and Real-World Applications
The practical implementation of ML Ops demonstrates its transformative impact on various industries.
Case Study 1: Financial Services Industry
In the financial sector, a leading bank implemented ML Ops to manage risk assessment models. The bank utilized ML Ops for "Continuous integration in machine learning," significantly reducing the time to deploy models from months to weeks. This enhancement not only boosted "AI operational efficiency" but also improved the accuracy of risk predictions, demonstrating the "Role of ML Ops in enhancing machine learning workflows."
Case Study 2: Healthcare Sector
A healthcare analytics firm adopted ML Ops to optimize the development and deployment of predictive models for patient care. By leveraging "Data science automation" and "AI model deployment," the firm achieved a streamlined process for updating and managing models, ensuring high reliability and accuracy in patient data analysis. This case illustrates the "Machine learning process improvements using ML Ops," enhancing patient outcomes through efficient data handling.
Case Study 3: Retail and E-commerce
An e-commerce giant integrated ML Ops to manage its recommendation systems. The adoption of "Automated machine learning workflows" and "ML model management" allowed for real-time updating and optimization of recommendation engines, leading to increased customer satisfaction and sales. This case study highlights the "ML Ops Benefits" in a dynamic, data-intensive environment.
Case Study 4: Autonomous Vehicle Development
In the field of autonomous vehicles, a tech company used ML Ops for developing and testing AI models. By applying "Machine learning scalability" and "DevOps for machine learning," the company accelerated its development cycle, ensuring robust and safe AI systems for autonomous navigation. This application underscores the significance of ML Ops in high-stakes, rapidly evolving technological domains.
These case studies exemplify how ML Ops transforms machine learning lifecycles across various industries. By addressing "Challenges and Solutions in ML Ops Implementation," businesses can harness the full potential of ML Ops, ensuring "Machine Learning Processes" are more efficient, scalable, and aligned with organizational goals. These real-world applications serve as a testament to the versatility and impact of ML Ops in enhancing business operations and driving innovation.
The Future of ML Ops: Trends and Predictions
As we look towards the horizon of machine learning, the role of ML Ops in shaping its future becomes increasingly evident. This section explores the emerging trends and potential integrations of ML Ops, emphasizing how it could redefine Machine Learning Processes and enhance AI operational efficiency.
1. Integration with Advanced Technologies:
A significant trend is the integration of ML Ops with technologies like Artificial Intelligence (AI) and the Internet of Things (IoT). This synergy is expected to enhance Machine learning scalability and enable more sophisticated Data science automation. For instance, combining ML Ops with AI can lead to more efficient model training and deployment, while IoT integration could revolutionize real-time data processing and analysis.
2. Enhanced Automation and Efficiency:
The push towards more automated and efficient workflows is at the forefront of ML Ops evolution. Advanced tools and platforms are being developed to streamline Automated machine learning workflows and Data orchestration in ML, aiming to minimize human intervention and reduce the time from model development to deployment.
3. Focus on Model Management and Deployment:
ML model management and AI model deployment are set to become more refined and robust. Tools that offer better version control, model tracking, and performance monitoring are expected to become standard in ML Ops, facilitating smoother "Continuous integration in machine learning."
4. Scalability and Flexibility:
As machine learning applications grow in complexity and size, "Machine learning scalability" remains a key focus area. ML Ops is predicted to evolve in ways that support scalable architectures, enabling machine learning models to handle larger datasets and more complex computations without compromising performance.
5. Emphasis on Security and Compliance:
Security and regulatory compliance are increasingly critical, especially with growing concerns around data privacy. Future ML Ops solutions will likely incorporate enhanced security features and compliance checks, ensuring that machine learning practices align with legal and ethical standards.
6. Cross-Domain Applications:
ML Ops is expected to expand beyond traditional tech domains, finding applications in diverse fields such as healthcare, finance, and manufacturing. This expansion will demonstrate the versatility of ML Ops in optimizing machine learning across various sectors.
7. Collaboration and Open-Source Tools:
Collaboration between industry and academia, along with the growing popularity of open-source ML Ops tools, will drive innovation and accessibility in this field. This collaboration could lead to breakthroughs in "DevOps for machine learning," making advanced ML Ops tools more accessible to a broader range of users.
Challenges and Solutions in ML Ops Implementation
Implementing ML Ops in machine learning lifecycles presents unique challenges, yet offers transformative solutions. This section addresses the common hurdles encountered and the best practices for overcoming them, leveraging keywords like "Machine learning pipeline optimization" and "Data orchestration in ML."
1. Integration Complexity:
One of the primary challenges is integrating ML Ops into existing machine learning systems. This process often requires significant adjustments in both infrastructure and workflow. Solutions include adopting modular ML Ops frameworks that allow gradual integration and focusing on "Continuous integration in machine learning" to streamline the process.
2. Data Management Issues:
Effective "Data orchestration in ML" is crucial. Challenges here include managing large volumes of data and ensuring data quality. Solutions involve implementing robust data management systems and utilizing automation tools for efficient "Data science automation."
3. Scalability and Performance:
As machine learning applications grow, "Machine learning scalability" becomes a critical concern. To address this, ML Ops solutions should be designed with scalability in mind, using cloud-based services or distributed computing environments to handle increased workloads.
4. Security and Compliance:
Security breaches and compliance with regulations like GDPR pose significant challenges. Adopting a security-first approach in ML Ops, including regular security audits and compliance checks, can mitigate these risks.
5. Skill Gap:
A lack of expertise in ML Ops can hinder its implementation. Overcoming this challenge involves investing in training and development for existing teams or hiring specialized personnel. Incorporating "Automated machine learning workflows" can also reduce the burden on teams.
6. Model Deployment and Monitoring:
Challenges in "AI model deployment" include ensuring model accuracy and performance post-deployment. Solutions include setting up robust monitoring systems for real-time performance tracking and employing "ML model management" tools for easy deployment and version control.
7. Continuous Improvement:
Finally, evolving machine learning models require continuous updating and improvement. Implementing agile methodologies in ML Ops, focusing on AI operational efficiency, and utilizing DevOps practices can foster a culture of continuous improvement and innovation.
Final Thoughts
In summary, ML Ops has emerged as a pivotal force in driving efficiency and optimization in machine learning processes. This article has explored various facets of ML Ops, highlighting its critical role in enhancing "Machine Learning Efficiency" and providing key benefits in the realms of "Machine Learning Processes" and "ML Operationalization."
Through our exploration, we've uncovered the essential aspects of ML Ops that make it indispensable in modern business environments:
- Enhancing Efficiency: ML Ops significantly boosts efficiency in machine learning workflows, as underscored by our analysis of "Improving efficiency in machine learning with ML Ops."
- Optimizing Processes: The role of ML Ops in "Optimizing Machine Learning" is undeniable, with its capabilities in automating and streamlining complex machine learning lifecycles.
- Scalability and Deployment: Its impact on "AI model deployment" and "Machine learning scalability" positions ML Ops as a cornerstone in scalable, robust AI applications.
- Technical Advancements: The article's technical deep-dive illuminated how ML Ops integrates "Data science automation" and "Continuous integration in machine learning," ensuring advanced operational capabilities.
This discourse underscores the transformative power of ML Ops in reshaping machine learning, aligning with the latest trends and predictions. Its ability to address and overcome various challenges — from "Machine learning pipeline optimization" to "Data orchestration in ML" — further cements its role as a game-changer in the AI and machine learning landscape.
Need Expert Guidance?
For businesses looking to harness the full potential of ML Ops and navigate the complexities of implementing these solutions, expert guidance is crucial. Antematter offers specialized consultation and services in ML Ops. Our team of skilled engineers is adept at tailoring ML Ops solutions to meet your specific business needs and objectives. Discover how our expertise can help optimize your machine learning processes by visiting Antematter.